How To Find The Sampling Distribution Of Xbar
six.two: The Sampling Distribution of the Sample Mean
- Folio ID
- 570
Learning Objectives
- To learn what the sampling distribution of \(\overline{X}\) is when the sample size is big.
- To larn what the sampling distribution of \(\overline{X}\) is when the population is normal.
In Example 6.1.i, we constructed the probability distribution of the sample mean for samples of size two drawn from the population of four rowers. The probability distribution is:
\[\begin{array}{c|c c c c c c c} \bar{x} & 152 & 154 & 156 & 158 & 160 & 162 & 164\\ \hline P(\bar{x}) &\dfrac{1}{16} &\dfrac{ii}{16} &\dfrac{3}{xvi} &\dfrac{four}{16} &\dfrac{3}{16} &\dfrac{2}{16} &\dfrac{one}{16}\\ \stop{array}\]
Figure \(\PageIndex{1}\) shows a side-by-side comparison of a histogram for the original population and a histogram for this distribution. Whereas the distribution of the population is uniform, the sampling distribution of the mean has a shape approaching the shape of the familiar bell curve. This phenomenon of the sampling distribution of the hateful taking on a bell shape even though the population distribution is non bong-shaped happens in general. Here is a somewhat more realistic instance.
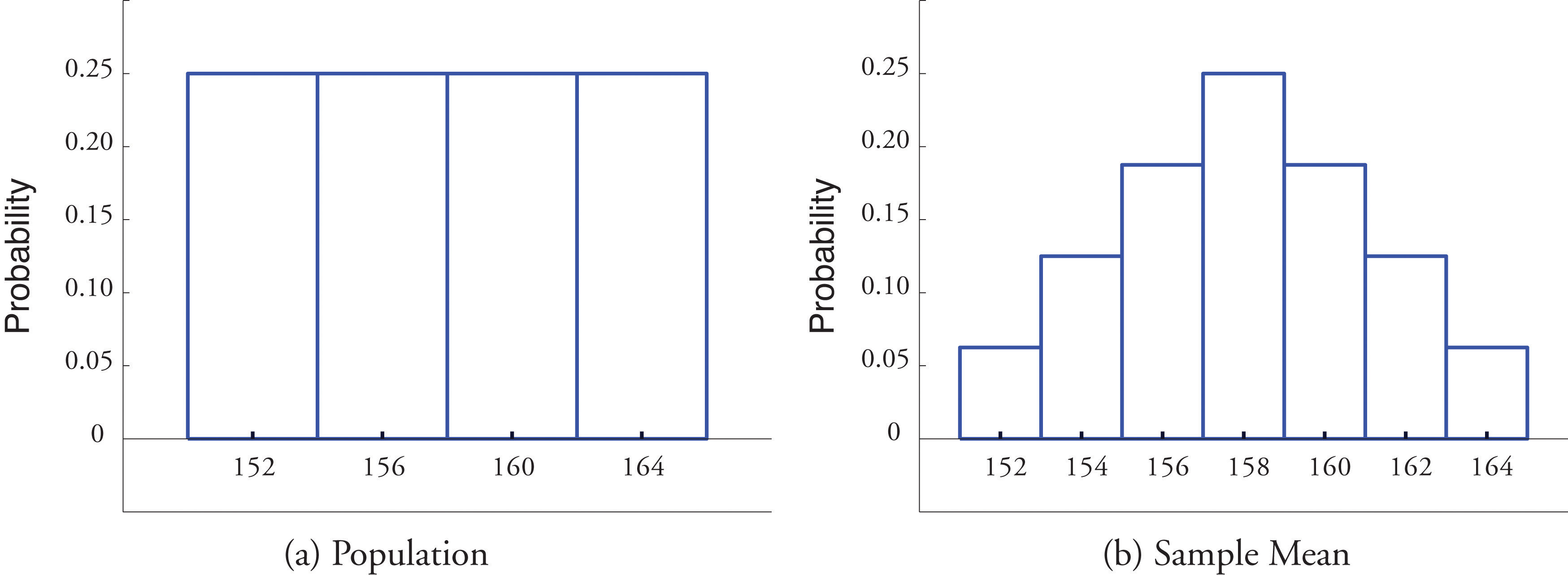
Suppose we take samples of size \(1\), \(v\), \(10\), or \(20\) from a population that consists entirely of the numbers \(0\) and \(1\), one-half the population \(0\), half \(one\), so that the population mean is \(0.5\). The sampling distributions are:
\(n = 1\):
\[\begin{array}{c|c c } \bar{x} & 0 & one \\ \hline P(\bar{x}) &0.5 &0.five \\ \end{assortment} \nonumber\]
\(due north = 5\):
\[\begin{array}{c|c c c c c c} \bar{ten} & 0 & 0.ii & 0.iv & 0.half dozen & 0.8 & one \\ \hline P(\bar{x}) &0.03 &0.16 &0.31 &0.31 &0.16 &0.03 \\ \stop{assortment} \nonumber\]
\(due north = 10\):
\[\begin{assortment}{c|c c c c c c c c c c c} \bar{x} & 0 & 0.i & 0.ii & 0.3 & 0.four & 0.5 & 0.6 & 0.7 & 0.8 & 0.nine & 1 \\ \hline P(\bar{10}) &0.00 &0.01 &0.04 &0.12 &0.21 &0.25 &0.21 &0.12 &0.04 &0.01 &0.00 \\ \end{array} \nonumber\]
\(north = 20\):
\[\begin{array}{c|c c c c c c c c c c c} \bar{10} & 0 & 0.05 & 0.x & 0.fifteen & 0.twenty & 0.25 & 0.30 & 0.35 & 0.xl & 0.45 & 0.50 \\ \hline P(\bar{x}) &0.00 &0.00 &0.00 &0.00 &0.00 &0.01 &0.04 &0.07 &0.12 &0.16 &0.18 \\ \end{array} \nonumber\]
and
\[\begin{assortment}{c|c c c c c c c c c c } \bar{x} & 0.55 & 0.60 & 0.65 & 0.lxx & 0.75 & 0.eighty & 0.85 & 0.90 & 0.95 & 1 \\ \hline P(\bar{x}) &0.16 &0.12 &0.07 &0.04 &0.01 &0.00 &0.00 &0.00 &0.00 &0.00 \\ \terminate{assortment} \nonumber\]
Histograms illustrating these distributions are shown in Figure \(\PageIndex{two}\).
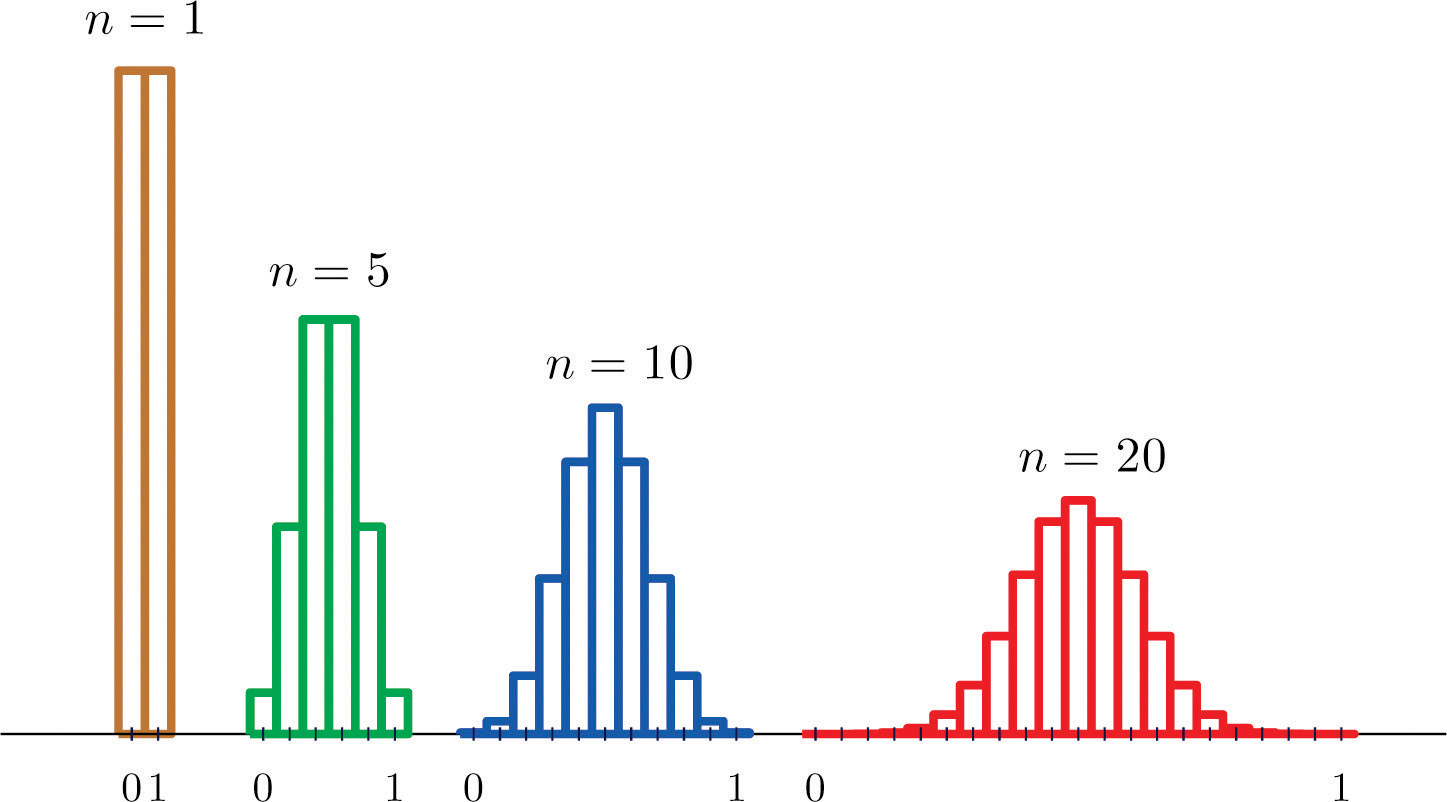
As \(due north\) increases the sampling distribution of \(\overline{10}\) evolves in an interesting way: the probabilities on the lower and the upper ends shrink and the probabilities in the heart become larger in relation to them. If we were to continue to increment \(n\) then the shape of the sampling distribution would go smoother and more than bong-shaped.
What we are seeing in these examples does not depend on the detail population distributions involved. In full general, one may starting time with whatever distribution and the sampling distribution of the sample mean volition increasingly resemble the bell-shaped normal curve as the sample size increases. This is the content of the Cardinal Limit Theorem.
The Central Limit Theorem
For samples of size \(30\) or more, the sample hateful is approximately unremarkably distributed, with mean \(\mu _{\overline{X}}=\mu\) and standard departure \(\sigma _{\overline{X}}=\dfrac{\sigma }{\sqrt{n}}\), where \(due north\) is the sample size. The larger the sample size, the improve the approximation. The Central Limit Theorem is illustrated for several common population distributions in Figure \(\PageIndex{3}\).
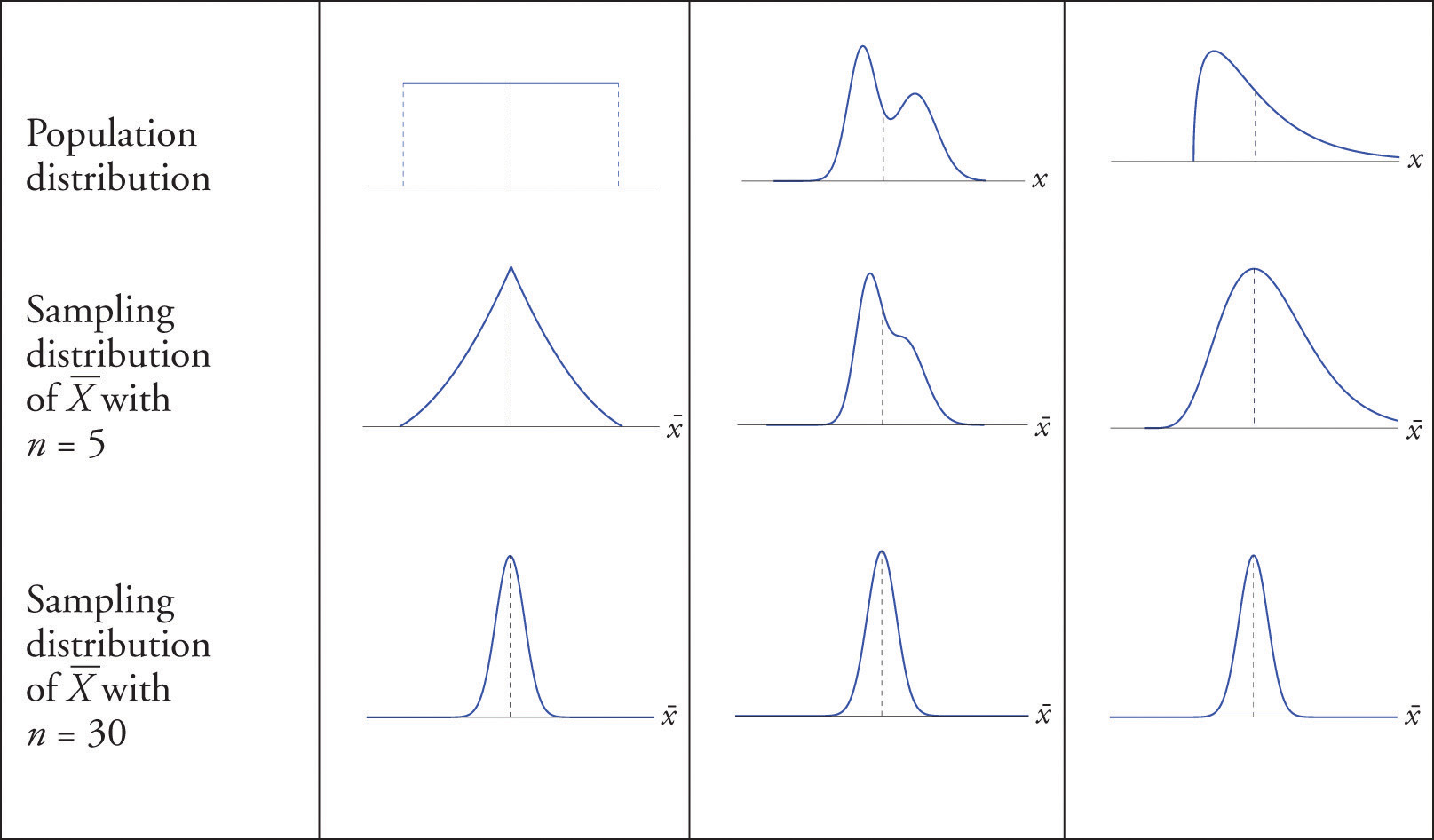
The dashed vertical lines in the figures locate the population mean. Regardless of the distribution of the population, every bit the sample size is increased the shape of the sampling distribution of the sample mean becomes increasingly bong-shaped, centered on the population mean. Typically by the time the sample size is \(30\) the distribution of the sample mean is practically the same every bit a normal distribution.
The importance of the Fundamental Limit Theorem is that it allows u.s. to make probability statements virtually the sample mean, specifically in relation to its value in comparison to the population mean, as nosotros volition see in the examples. But to apply the result properly nosotros must outset realize that there are two split random variables (and therefore two probability distributions) at play:
- \(X\), the measurement of a unmarried element selected at random from the population; the distribution of \(X\) is the distribution of the population, with hateful the population mean \(\mu\) and standard deviation the population standard deviation \(\sigma\);
- \(\overline{Ten}\), the mean of the measurements in a sample of size \(n\); the distribution of \(\overline{X}\) is its sampling distribution, with mean \(\mu _{\overline{X}}=\mu\) and standard deviation \(\sigma _{\overline{10}}=\dfrac{\sigma }{\sqrt{north}}\).
Example \(\PageIndex{1}\)
Let \(\overline{X}\) be the hateful of a random sample of size \(l\) drawn from a population with hateful \(112\) and standard deviation \(40\).
- Find the mean and standard deviation of \(\overline{X}\).
- Find the probability that \(\overline{X}\) assumes a value between \(110\) and \(114\).
- Find the probability that \(\overline{Ten}\) assumes a value greater than \(113\).
Solution:
- By the formulas in the previous department \[\mu _{\overline{X}}=\mu=112 \nonumber\] and \[ \sigma_{\overline{Ten}}=\dfrac{\sigma}{\sqrt{n}}=\dfrac{40} {\sqrt{fifty}}=5.65685 \nonumber\]
- Since the sample size is at least \(30\), the Central Limit Theorem applies: \(\overline{Ten}\) is approximately unremarkably distributed. We compute probabilities using Figure five.iii.ane in the usual way, but beingness conscientious to use \(\sigma _{\overline{X}}\) and not \(\sigma\) when we standardize:
\[\brainstorm{align*} P(110<\overline{X}<114)&= P\left ( \dfrac{110-\mu _{\overline{X}}}{\sigma _{\overline{X}}} <Z<\dfrac{114-\mu _{\overline{X}}}{\sigma _{\overline{10}}}\right )\\[4pt] &= P\left ( \dfrac{110-112}{v.65685} <Z<\dfrac{114-112}{five.65685}\right )\\[4pt] &= P(-0.35<Z<0.35)\\[4pt] &= 0.6368-0.3632\\[4pt] &= 0.2736 \end{align*}\]
- Similarly
\[\brainstorm{align*} P(\overline{10}> 113)&= P\left ( Z>\dfrac{113-\mu _{\overline{X}}}{\sigma _{\overline{X}}}\right )\\[4pt] &= P\left ( Z>\dfrac{113-112}{5.65685}\right )\\[4pt] &= P(Z>0.18)\\[4pt] &= one-P(Z<0.18)\\[4pt] &= one-0.5714\\[4pt] &= 0.4286 \stop{align*}\]
Note that if in the above example we had been asked to compute the probability that the value of a single randomly selected element of the population exceeds \(113\), that is, to compute the number \(P(Ten>113)\), we would non have been able to do then, since we do not know the distribution of \(X\), but merely that its mean is \(112\) and its standard deviation is \(twoscore\). By contrast nosotros could compute \(P(\overline{X}>113)\) even without consummate knowledge of the distribution of \(10\) because the Central Limit Theorem guarantees that \(\overline{10}\) is approximately normal.
Example \(\PageIndex{2}\)
The numerical population of form indicate averages at a college has mean \(two.61\) and standard deviation \(0.5\). If a random sample of size \(100\) is taken from the population, what is the probability that the sample mean will be between \(2.51\) and \(2.71\)?
Solution:
The sample mean \(\overline{X}\) has mean \(\mu _{\overline{X}}=\mu =2.61\) and standard deviation \(\sigma _{\overline{X}}=\dfrac{\sigma }{\sqrt{n}}=\dfrac{0.5}{ten}=0.05\), so
\[\begin{align*} P(2.51<\overline{10}<2.71)&= P\left ( \dfrac{2.51-\mu _{\overline{X}}}{\sigma _{\overline{X}}} <Z<\dfrac{2.71-\mu _{\overline{X}}}{\sigma _{\overline{X}}}\right )\\[4pt] &= P\left ( \dfrac{2.51-2.61}{0.05} <Z<\dfrac{two.71-2.61}{0.05}\correct )\\[4pt] &= P(-2<Z<2)\\[4pt] &= P(Z<two)-P(Z<-ii)\\[4pt] &= 0.9772-0.0228\\[4pt] &= 0.9544 \end{marshal*}\]
Unremarkably Distributed Populations
The Central Limit Theorem says that no matter what the distribution of the population is, as long as the sample is "large," significant of size \(30\) or more, the sample hateful is approximately normally distributed. If the population is normal to brainstorm with then the sample mean as well has a normal distribution, regardless of the sample size.
For samples of whatsoever size drawn from a unremarkably distributed population, the sample hateful is normally distributed, with mean \(μ_X=μ\) and standard departure \(σ_X =σ/\sqrt{n}\), where \(n\) is the sample size.
The outcome of increasing the sample size is shown in Figure \(\PageIndex{4}\).
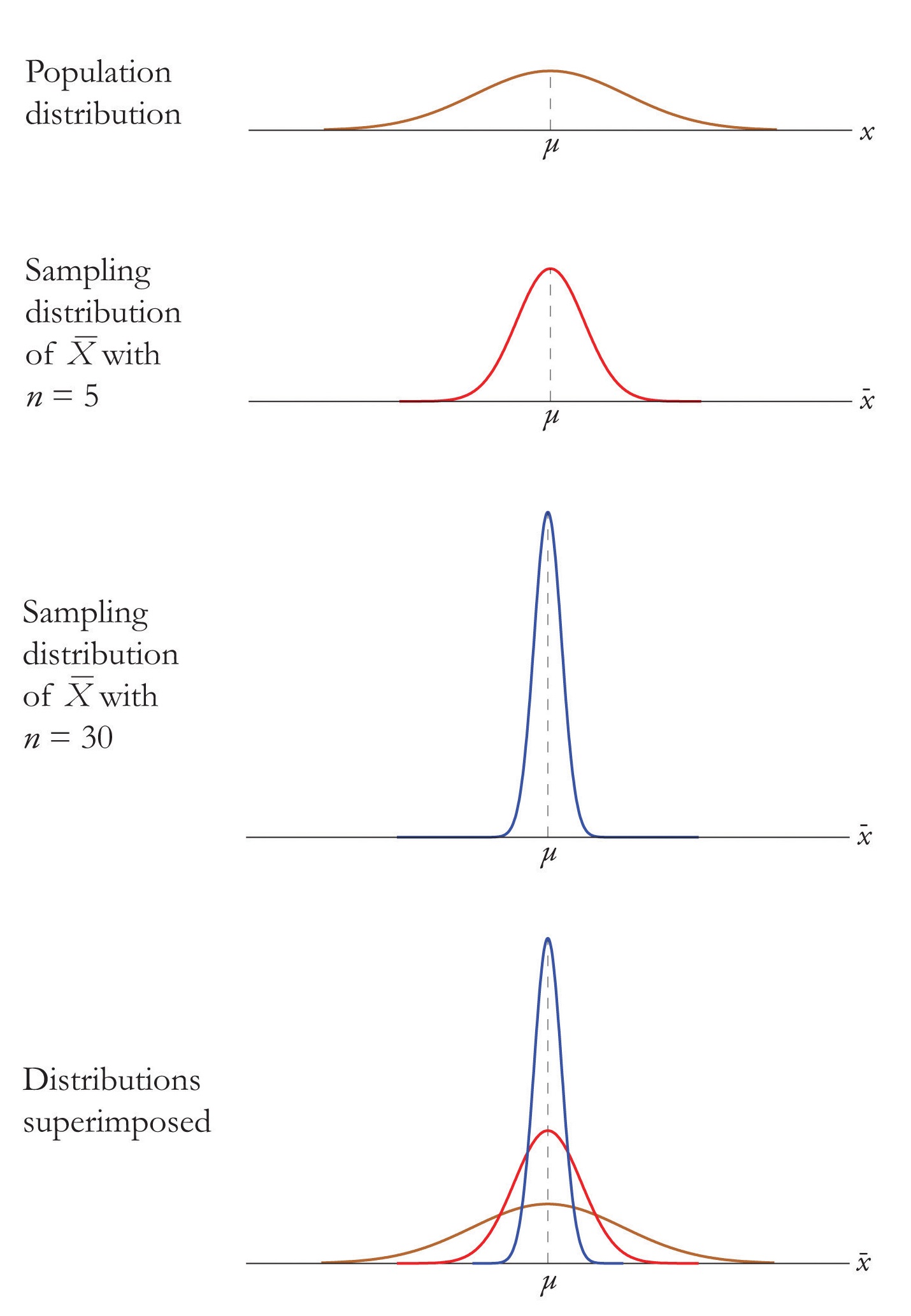
Example \(\PageIndex{three}\)
A prototype automotive tire has a blueprint life of \(38,500\) miles with a standard deviation of \(2,500\) miles. Five such tires are manufactured and tested. On the assumption that the actual population mean is \(38,500\) miles and the actual population standard difference is \(2,500\) miles, find the probability that the sample mean will exist less than \(36,000\) miles. Assume that the distribution of lifetimes of such tires is normal.
Solution:
For simplicity nosotros use units of thousands of miles. And so the sample mean \(\overline{X}\) has mean \(\mu _{\overline{X}}=\mu =38.5\) and standard deviation \(\sigma _{\overline{Ten}}=\dfrac{\sigma }{\sqrt{north}}=\dfrac{2.5}{\sqrt{5}}=1.11803\). Since the population is normally distributed, then is \(\overline{Ten}\), hence
\[\begin{align*} P(\overline{X}<36)&= P\left ( Z<\dfrac{36-\mu _{\overline{Ten}}}{\sigma _{\overline{Ten}}}\right )\\[4pt] &= P\left ( Z<\dfrac{36-38.5}{ane.11803}\correct )\\[4pt] &= P(Z<-2.24)\\[4pt] &= 0.0125 \end{marshal*}\]
That is, if the tires perform equally designed, there is but about a \(1.25\%\) chance that the average of a sample of this size would be then low.
Example \(\PageIndex{4}\)
An automobile battery manufacturer claims that its midgrade bombardment has a mean life of \(fifty\) months with a standard difference of \(six\) months. Suppose the distribution of battery lives of this particular brand is approximately normal.
- On the assumption that the manufacturer'south claims are true, find the probability that a randomly selected bombardment of this type will last less than \(48\) months.
- On the same assumption, find the probability that the mean of a random sample of \(36\) such batteries will be less than \(48\) months.
Solution:
- Since the population is known to take a normal distribution
\[\begin{align*} P(X<48)&= P\left ( Z<\dfrac{48-\mu }{\sigma }\right )\\[4pt] &= P\left ( Z<\dfrac{48-l}{vi}\right )\\[4pt] &= P(Z<-0.33)\\[4pt] &= 0.3707 \terminate{align*}\]
- The sample hateful has mean \(\mu _{\overline{X}}=\mu =50\) and standard departure \(\sigma _{\overline{10}}=\dfrac{\sigma }{\sqrt{n}}=\dfrac{six}{\sqrt{36}}=1\). Thus
\[\begin{align*} P(\overline{X}<48)&= P\left ( Z<\dfrac{48-\mu _{\overline{Ten}}}{\sigma _{\overline{Ten}}}\right )\\[4pt] &= P\left ( Z<\dfrac{48-50}{i}\right )\\[4pt] &= P(Z<-2)\\[4pt] &= 0.0228 \terminate{align*}\]
Primal Takeaway
- When the sample size is at least \(30\) the sample mean is normally distributed.
- When the population is normal the sample mean is usually distributed regardless of the sample size.
How To Find The Sampling Distribution Of Xbar,
Source: https://stats.libretexts.org/Bookshelves/Introductory_Statistics/Book%3A_Introductory_Statistics_(Shafer_and_Zhang)/06%3A_Sampling_Distributions/6.02%3A_The_Sampling_Distribution_of_the_Sample_Mean
Posted by: millsextre1971.blogspot.com
0 Response to "How To Find The Sampling Distribution Of Xbar"
Post a Comment